Oxford example
This tutorial demonstrates the lava.lib.dl.netx
api for running Oxford network trained using lava.lib.dl.slayer
. The training example can be found here here
The task is to learn to transform a random Poisson spike train to an output spike pattern that resembles The Radcliffe Camera building of Oxford University, England. The input and output both consist of 200 neurons each and the spikes span approximately 1900ms. The input and output pair are converted from SuperSpike (© GPL-3).
Input | Output |
[1]:
import numpy as np
import matplotlib.pyplot as plt
import logging
from lava.magma.core.run_configs import Loihi2HwCfg, Loihi2SimCfg
from lava.magma.core.run_conditions import RunSteps
from lava.proc import io
from utils import InputAdapter, OutputAdapter, OxfordMonitor
Network Exchange (NetX) Libarary
The NetX api allows automatic creation of Lava process from the network specification. It is available as a part of the lava-dl library as lava.lib.dl.netx
[2]:
from lava.lib.dl import netx
from lava.lib.dl import slayer
Import modules for Loihi2 execution
Check if Loihi2 compiker is available and import related modules.
[3]:
from lava.utils.system import Loihi2
Loihi2.preferred_partition = 'oheogulch'
loihi2_is_available = Loihi2.is_loihi2_available
if loihi2_is_available:
from lava.proc import embedded_io as eio
print(f'Running on {Loihi2.partition}')
else:
print("Loihi2 compiler is not available in this system. "
"This tutorial will execute on CPU backend.")
Running on kp_stack
Create network block
A lava process describing the network can be created by simply instantiating netx.hdf5.Network
with the path of the desired hdf5 network description file. * The input layer is accessible as net.in_layer
. * The output layer is accessible as net.out_layer
. * All the constituent layers are accessible as a list: net.layers
.
[4]:
net = netx.hdf5.Network(net_config='Trained/network.net')
print(net)
| Type | W | H | C | ker | str | pad | dil | grp |delay|
|Dense | 1| 1| 256| | | | | |False|
|Dense | 1| 1| 200| | | | | |False|
[5]:
print(f'There are {len(net)} layers in the network:')
for l in net.layers:
print(f'{l.block:5s} : {l.name:10s}, shape : {l.shape}')
There are 2 layers in network:
Dense : Process_1 , shape : (256,)
Dense : Process_4 , shape : (200,)
Create Spike Input/Ouptut process
Here, we will use RingBuffer
processes in lava.proc.io.{source/sink}
to generate spike that is sent to the network and record the spike output from the network.
There are 200 neurons and the input spikes span apprximately 2000 steps.
We will use slayer.io
utilities to read the event data and convert them to dense spike data.
[6]:
input = slayer.io.read_np_spikes('input.npy')
target = slayer.io.read_np_spikes('output.npy')
source = io.source.RingBuffer(data=input.to_tensor(dim=(1, 200, 2000)).squeeze())
sink = io.sink.RingBuffer(shape=net.out.shape, buffer=2000)
inp_adapter = InputAdapter(shape=net.inp.shape)
out_adapter = OutputAdapter(shape=net.out.shape)
source.s_out.connect(inp_adapter.inp)
inp_adapter.out.connect(net.inp)
net.out.connect(out_adapter.inp)
out_adapter.out.connect(sink.a_in)
Run the network
We will run the network for 2000 steps and read the network’s output.
Switching between Loihi 2 hardware and CPU simulation is as simple as changing the run configuration settings.
[7]:
if loihi2_is_available:
from utils import CustomHwRunConfig
run_config = CustomHwRunConfig()
else:
from utils import CustomSimRunConfig
run_config = CustomSimRunConfig()
run_condition = RunSteps(num_steps=2000)
net._log_config.level = logging.INFO
net.run(condition=run_condition, run_cfg=run_config)
output = sink.data.get()
net.stop()
Violation core_id=0 reg_name='SynMem' allocation=16128 self.cost_db.registers[reg_name]=12000
Violation core_id=1 reg_name='SynMem' allocation=16200 self.cost_db.registers[reg_name]=12000
Final max_ratio=2, Violation core_id=0 reg_name='SynMem' allocation=16128 self.cost_db.registers[reg_name]=12000
Final max_ratio=2, Violation core_id=1 reg_name='SynMem' allocation=16200 self.cost_db.registers[reg_name]=12000
Final max_ratio=2, Violation core_id=0 reg_name='SynMem' allocation=16128 self.cost_db.registers[reg_name]=12000
Final max_ratio=2, Violation core_id=1 reg_name='SynMem' allocation=16200 self.cost_db.registers[reg_name]=12000
Final max_ratio=2, Violation core_id=0 reg_name='SynMem' allocation=16128 self.cost_db.registers[reg_name]=12000
Final max_ratio=2, Violation core_id=1 reg_name='SynMem' allocation=16200 self.cost_db.registers[reg_name]=12000
Final max_ratio=2, Violation core_id=0 reg_name='SynMem' allocation=16128 self.cost_db.registers[reg_name]=12000
Final max_ratio=2, Violation core_id=1 reg_name='SynMem' allocation=16000 self.cost_db.registers[reg_name]=12000
Final max_ratio=2, Per core distribution:
----------------------------------------------------------------
| AxonIn |NeuronGr| Neurons|Synapses| AxonMap| AxonMem| Cores |
|--------------------------------------------------------------|
| 256| 1| 100| 8064| 100| 0| 2|
| 200| 1| 85| 5400| 85| 0| 3|
|--------------------------------------------------------------|
| Total | 5|
----------------------------------------------------------------
INFO:DRV: SLURM is being run in background
INFO:DRV: Connecting to 10.54.73.72:41019
INFO:DRV: Host server up..............Done 0.49s
INFO:DRV: Mapping chipIds.............Done 0.02ms
INFO:DRV: Mapping coreIds.............Done 0.07ms
INFO:DRV: Partitioning neuron groups..Done 1.38ms
INFO:DRV: Mapping axons...............Done 2.52ms
INFO:DRV: Partitioning MPDS...........Done 0.61ms
INFO:DRV: Creating Embedded Snips and ChannelsDone 0.02s
INFO:DRV: Compiling Embedded snips....Done 2.90s
INFO:DRV: Compiling Host snips........Done 0.06ms
INFO:DRV: Compiling Register Probes...Done 0.09ms
INFO:DRV: Compiling Spike Probes......Done 0.01ms
INFO:HST: Args chip=0 cpu=0 /home/sshresth/lava-nc/frameworks.ai.nx.nxsdk/nxcore/arch/base/pre_execution/../../../../temp/27a4c4ec-3a0e-11ed-bc43-dd9a32d49015/launcher_chip0_lmt0.bin --chips=1 --remote-relay=0
INFO:HST: Args chip=0 cpu=1 /home/sshresth/lava-nc/frameworks.ai.nx.nxsdk/nxcore/arch/base/pre_execution/../../../../temp/27a4c4ec-3a0e-11ed-bc43-dd9a32d49015/launcher_chip0_lmt1.bin --chips=1 --remote-relay=0
INFO:HST: Nx...
INFO:DRV: Booting up..................Done 1.57s
INFO:DRV: Encoding probes.............Done 0.01ms
INFO:DRV: Transferring probes.........Done 7.08ms
INFO:DRV: Configuring registers.......Done 0.61s
INFO:DRV: Transferring spikes.........Done 0.00ms
INFO:HST: chip=0 msg=00018114 00ffff00
INFO:DRV: Executing...................Done 16.09s
INFO:DRV: Processing timeseries.......Done 0.01ms
INFO:DRV: Executor: 2000 timesteps........Done 16.85s
INFO:HST: Execution has not started yet or has finished.
INFO:HST: Stopping Execution : at 2000
INFO:HST: chip=0 cpu=1 halted, status=0x0
INFO:HST: chip=0 cpu=0 halted, status=0x0
Plot the results
Finally, convert output spike data into an event and plot them.
[8]:
out_event = slayer.io.tensor_to_event(output.reshape((1,) + output.shape))
gt_event = slayer.io.read_np_spikes('ground_truth.npy')
[9]:
fig, ax = plt.subplots(1, 2, figsize=(15, 7))
ax[0].plot(gt_event.t, gt_event.x, '.', markersize=2)
ax[1].plot(out_event.t, out_event.x, '.', markersize=2)
ax[0].set_title('Slayer Reference')
ax[0].set_ylabel('Neuron ID')
ax[0].set_xlabel('time')
ax[1].set_title('Lava')
ax[1].set_xlabel('time')
[9]:
Text(0.5, 0, 'time')
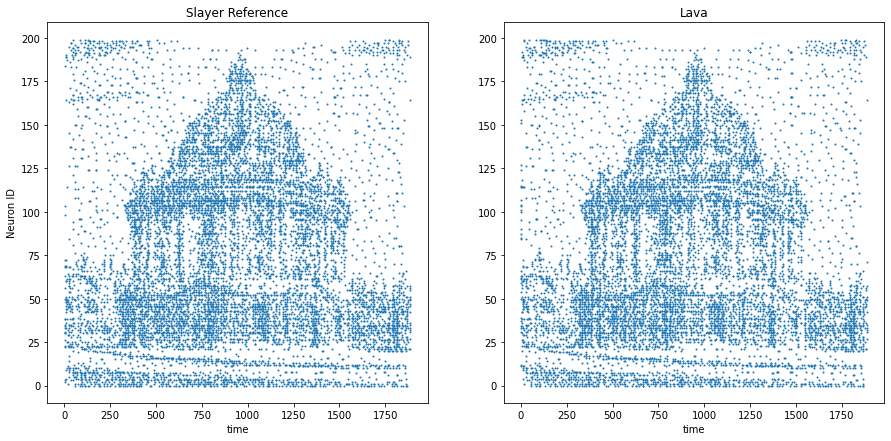